1.
A matrix may contain numeric values only.
2.
A matrix must not be singular.
3.
A data frame may contain variables that have different modes.
4.
A data frame may contain variables of different lengths.
Q 1 / 52
`unclass(as.Date("1971-01-01"))`
1.
1
2.
365
3.
4
4.
12
Q 2 / 52
1.
remove()
2.
erase()
3.
detach()
4.
delete()
Q 3 / 52
xvect<-c(1,2,3) xvect[2] <- "2" xvect
1.
[1
2.
[1
3.
[1
4.
[1
Q 4 / 52
1.
`height(length(height))`
2.
`height[length(height)]`
3.
`height[length[height]]`
4.
`height(5)`
Q 5 / 52

1.
sample with replacement
2.
population
3.
trimmed sample
4.
sample <-- not sure
Q 6 / 52
1.
`Var_A!`
2.
`_VarA`
3.
`.2Var_A`
4.
`Var2_A`
Q 7 / 52
1.
A matrix has two dimensions, while an array can have three or more dimensions.
2.
An array is a subtype of the data frame, while a matrix is a separate type entirely.
3.
A matrix can have columns of different lengths, but an array's columns must all be the same length.
4.
A matrix may contain numeric values only, while an array can mix different types of values.
Q 8 / 52
1.
type
2.
length
3.
attributes
4.
scalar
Q 9 / 52

1.
StDf[1:2,-3]
2.
StDf[1:2,1]
3.
StDf[1:2,]
4.
StDf[1,2,]
Q 10 / 52
1.
BOF(pizza, 5)
2.
first(pizza, 5)
3.
top(pizza, 5)
4.
head(pizza, 5)
Q 11 / 52
1.
console(-25)
2.
console(reverse=TRUE)
3.
history()
4.
history(max.show = 25)
Q 12 / 52
> class( d.pizza[ , "temperature" ] ) > "numeric"
1.
`class( d.pizza( , "temperature" ) )`
2.
`class( d.pizza[ , "temperature"
3.
`class( d.pizza$temperature )`
4.
`class( d.pizza[ , "temperature", drop=F
Q 13 / 52
a <- c(3,3,6.5,8) b <- c(7,2,5.5,10) c <- a < b
1.
[1
2.
[1
3.
[1
4.
[1
Q 14 / 52
> y <- 1:9 > dim(y) <- c(3,3)
1.
No, y's new class is "array".
2.
Yes, y's new class is "matrix".
3.
No, y's new class is "vector".
4.
Yes, y's new class is "integer".
Q 15 / 52
`mydf <- data.frame(x=1:3, y=c("a","b","c"), stringAsFactors=FALSE)`
1.
list
2.
string
3.
factor
4.
character vector
Q 16 / 52
1.
Vectors are used only for numeric data, while lists are useful for both numeric and string data.
2.
Vectors and lists are the same thing and can be used interchangeably.
3.
A vector contains items of a single data type, while a list can contain items of different data types.
4.
Vectors are like arrays, while lists are like data frames.
Q 17 / 52
1.
list.objects()
2.
print.objects()
3.
getws()
4.
ls()
Q 18 / 52
1.
rbind()
2.
cbind()
3.
bind()
4.
coerce()
Q 19 / 52
1 mylist <- list(1,2,"C",4,5) 2 unlist(mylist)
1.
[1
2.
"C"
3.
[1
4.
[1
Q 20 / 52
x <- NA y <- x/1
1.
Inf
2.
Null
3.
NaN
4.
NA
Q 21 / 52
1.
`cor.test(Var1 ~ Var2)`
2.
`cor.test(mydata$(Var1,Var2))`
3.
`cor.test(mydata$Var1,mydata$Var2)`
4.
`cor.test(Var1,Var2, mydata)`
Q 22 / 52
library(DescTools) deliver <- aggregate(count,by=list(area,driver), FUN=mean) print(deliver)
1.
`attach(d.pizza)`
2.
`summarize(deliver)`
3.
`mean <- rbind(d.pizza,count)`
4.
`deliver[!complete.cases(deliver),]`
Q 23 / 52
1.
data frame: names() and rownames() matrix: colnames() and row.names()
2.
data frame: names() and row.names() matrix: dimnames() (not sure)
3.
data frame: colnames() and row.names() matrix: names() and rownames()
4.
data frame: colnames() and rownames() matrix: names() and row.names()
Q 24 / 52
v1<-list(1,2,3) v2<-list(c(4,5,6)) vbound<-cbind(v1,v2) v1<-c(1,2,3) v2<-list(4,5,6)) vbound<-cbind(v1,v2) v1<-c(1,2,3) v2<-c(4,5,6)) vbound<-cbind(v1,v2)
1.
v1<-list(1,2,3)
v2<-list(c(4,5,6))
vbound<-cbind(v1,v2)
2.
v1<-c(1,2,3)
v2<-list(4,5,6))
vbound<-cbind(v1,v2)
3.
v1<-c(1,2,3)
v2<-c(4,5,6))
vbound<-cbind(v1,v2)
Q 25 / 52
`Cpeople <- ournames %in% grep("^C", ournames, value=TRUE)`
1.
records where the first character is a C
2.
any record with a value containing a C
3.
TRUE or FALSE, depending on whether any character in ournames is C
4.
TRUE and FALSE values, depending on whether the first character in an ournames record is C
Q 26 / 52
v <- 1:3 names(v) <- c("a", "b", "c") v[4] <- 4
1.
""
2.
d
3.
NULL
4.
NA
Q 27 / 52
x <- c(1, 2, 3, 4) Output: [1] 2 3 4
1.
x[c(2, 3, 4)]
2.
x[-1]
3.
x[c(-1, 0, 0, 0)]
4.
x[c(-1, 2, 3, 4)]
Q 28 / 52
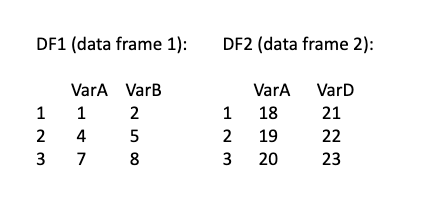
1.
6
2.
9
3.
3
4.
0
Q 29 / 52
x<-5:8 names(x)<-letters[5:8] x "5" "6" "7" "8" 5 6 7 8
1.
e f g h
2.
5 6 7 8
3.
e f g h
4.
e f g h
Q 30 / 52
x<-as.Date("2018-10-01")
1.
attr()
2.
months(x)
3.
as.month(x)
4.
month(x)
Q 31 / 52
fact<-factor(c("Rep","Dem","Dem","Rep")) fact Levels: Rep Dem fact[2]<-"Ind"
1.
>
2.
[,2]Ind
3.
invalid factor level, NA generated
4.
Ind
Q 32 / 52
StartDate<- as.Date("2020/2/28") StopDate<- as.Date("2020/3/1") StopDate-StartDate
1.
"1970-01-02"
2.
time difference of one day
3.
time difference of two days
4.
error in x-y: nonnumeric argument to binary operator
Q 33 / 52
> mtrx <- matrix( c(3,5,8,4), nrow= 2,ncol=2,byrow=TRUE) > newmat <- mtrx * mtrx
1.
it transpose **mtrx**
2.
it premultiplies the current **netwmat** row by the **newmat** column.
3.
it returns the results of a matrix multiplication
4.
It squares each cell in **mtrx**
Q 34 / 52
1.
connect()
2.
concat()
3.
contact()
4.
c()
Q 35 / 52
1.
Rdefaults.site
2.
Renviron.site
3.
Rprofile.site
4.
Rstatus.site
Q 36 / 52
1.
**ncol(mdf)** equals **length(mdf)**.
2.
The number of rows must equals the number of columns.
3.
The legnth of any column in **mdf** may differ from any other column in **mdf**
4.
All columns must have the same data type.
Q 37 / 52
1.
vector(MyList, length = 7)
2.
coerce(MyList, nrows = 1)
3.
unlist(MyList)
4.
coerce(MyList, nrows = 7)
Q 38 / 52
1.
ANOVAData, anovadata
2.
VisitPCA, VarX
3.
VisitPCA, varx
4.
Xvar, Yvar
Q 39 / 52
r StDf[, -1]
1.
all but the first row and first column of StDf
2.
all but the final column of StDf
3.
all but the first column of StDf
4.
only the first column of StDf
Q 40 / 52
1.
file.list()
2.
file.select()
3.
file.choose()
4.
file.open()
Q 41 / 52
1.
Each is a type of data frame.
2.
Each is a type of atomic vector.
3.
Each is a type of complex vector.
4.
Each is a type of raw vector.
Q 42 / 52
r MyMat = matrix(c(7, 9, 8, 6, 10, 12),nrow=2,ncol=3, byrow = TRUE)
1.
[ ,3]
[1, ] 8
[2, ] 12
2.
[1] 8 12
3.
[1] 10 12
4.
[ ,3]
[1, ] 10
[2, ] 12
Q 43 / 52
1.
the probability of making a Type I error
2.
the probability of not making a Type II error
3.
the probability of making a Type II error
4.
the probability of not making a Type I error
Q 44 / 52
r result <- lm(outcome ~ covariate + factor + covariate:factor, data = testcoef)
1.
It forces the intercepts of the individual regressions to zero.
2.
It calls for the effect of the covariate within each level of the factor.
3.
It calls for the effect of each variable from covariate to factor in testcoef.
4.
It forces the covariate to enter the equation before the factor levels.
Q 45 / 52
1.
integers and real values
2.
integers, real, and raw values
3.
real values only
4.
integers, real, and logical values
Q 46 / 52
1.
property
2.
integer
3.
number
4.
variant
Q 47 / 52
1.
`Rmat[upper.tri(Rmat)]`
2.
`upper.triangular(Rmat)`
3.
`upper.tri(Rmat)`
4.
`upper.diag(Rmat)`
Q 48 / 52
`x <- c(12L, 6L, 10L, 8L, 15L, 14L, 19L, 18L, 23L, 59L)`
1.
numeric
2.
integer
3.
single
4.
double
Q 49 / 52
`a <- list("10", TRUE, 5.6)`
1.
is.list(a[1])
2.
is.numeric(a[1])
3.
is.logical(a[1])
4.
is.character(a[1])
Q 50 / 52
`x <- as.Date("2018-10-01")` ### Q51. How do you obtain the row numbers in a data frame named `pizza` for which the value of `pizza$delivery_min` is greater than or equal to 30? late_delivery <- pizza$delivery_min >= 30 index_late <- index(late_delivery) index_late late_delivery <- pizza$delivery_min >= 30 rownum_late <- rownum(late_delivery) rownum_late late_delivery <- pizza$delivery_min >= 30 which_late <- which(late_delivery) which_late late_delivery <- pizza$delivery_min >= 30 late <- pizaa$late_delivery pizza$late
1.
late_delivery <- pizza$delivery_min >= 30
index_late <- index(late_delivery)
index_late
2.
late_delivery <- pizza$delivery_min >= 30
rownum_late <- rownum(late_delivery)
rownum_late
3.
late_delivery <- pizza$delivery_min >= 30
which_late <- which(late_delivery)
which_late
4.
late_delivery <- pizza$delivery_min >= 30
late <- pizaa$late_delivery
pizza$late
Q 51 / 52
`indat <- c("Ash Rd","Ash Cir","Ash St")`
1.
grepl("[Rd|Ave|Dr|St]", indat)
2.
grepl("Rd|Ave|Dr|St", indat)
3.
grepl("Rd,Ave,Dr,St", indat)
4.
grepl("[Rd],[Ave],[Dr],[St]", indat)
Q 52 / 52